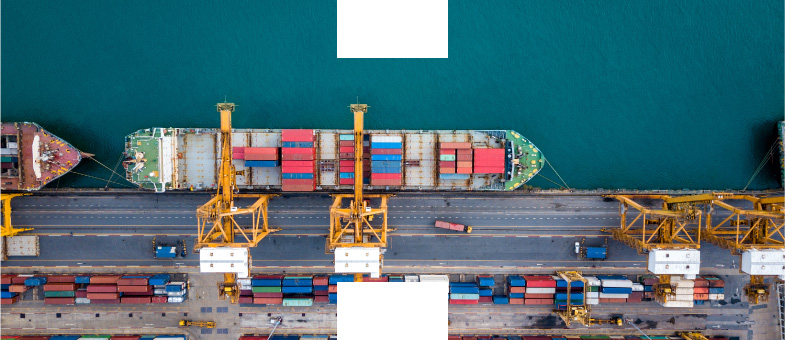
Why Dynamic Logistics Analytics for Supply Chains is More Important Than Ever
Try HeavyIQ Conversational Analytics on 400 million tweets
Download HEAVY.AI Free, a full-featured version available for use at no cost.
GET FREE LICENSEThe granular functions of the supply chain have typically flown under the radar for the average consumer. But over the course of the last two years, the general population has become all too familiar with the fragility and uncertainty of the supply chain. 2020 and 2021 produced a host of issues that created historically high supply chain disruptions. Covid–19 majorly impacted production and shipping in nearly every industry while demand continued to soar. The result? Everyone is paying more for pretty much everything, shipping times have increased drastically, and even some store shelves are bare.
In response, economic nationalism has crept in, and there is mounting pressure for companies to increase their employment and domestic production in higher-cost home markets, and to operate as efficiently as possible without raising prices or losing their competitive edge. To accomplish this, companies need to invest in proactive supply chain efficiency solutions that use advanced logistics analytics technology to help decision makers examine their supply networks, identify their own vulnerabilities, and make better data-driven decisions. This is where dynamic data analytics in the logistics industry come into play.
Data analytics for logistics pertains to the analytical techniques used to manage the logistics of supply chain elements such as storage, sorting, packaging, production, delivery, and returns in the most timely and cost–effective manner, and to gain insight and extract value from the available data. Logistics analytics software helps companies cope with the sheer volume, velocity, and variety of big data analytics in logistics and supply chain management. Supply chain data is siloed and lacks end-to-end visibility, making supply chain optimization efforts critical. Supply chain analytics and business intelligence tools can help an organization reduce costs, improve business processes and margins, better understand risks, increase accuracy in planning, achieve the lean supply chain, and prepare for the future.
The 4 Different Types of Data Analytics in Logistics
What is the inner-workings of logistics analytics for supply chains? How does it actually work? Here’s a quick rundown of the nitty gritty:
- Descriptive Supply Chain Analytics: Descriptive analytics answers “What happened?” Using various statistical methods, we can answer supply chain queries such as, “How has inventory changed over the last 6 months?” or “What is the return on this particular investment?”
- Diagnostic Supply Chain Analytics: Diagnostics analytics tells us why something happened, like a shipment was late, or why competition is outperforming us.
- Predictive Supply Chain Logistics: Logistic regression in predictive analytics and predictive models use current data to predict a likely future outcome. Predictive analytics in the logistics industry may answer questions such as: “How will a trade war with a particular country impact the availability of certain goods?”
- Prescriptive Supply Chain Analytics: Using optimization or embedded decision logic, prescriptive analytics tells us what the next best move is. This includes elements such as adjusting shipping routes, increasing or decreasing orders for raw goods, or rescheduling a product launch.
Now let’s take a look at some of the impactful ways that data analytics for logistics supply chain management can help companies improve their forecasting abilities, their responsiveness to customers, and their efficiency. Read on to learn more about some of the specific ways that big data analytics for logistics can help improve operational efficiency.
Forecasting Supply Needs
Customers expect companies to anticipate their needs and predict their behavior. A combination of cognitive computing, artificial intelligence, and machine learning algorithms helps us do just that. Real-time supply chain analytics use real-time data to automatically divert inventory where it’s needed most, avoiding understocking and overstocking, and reducing waste and costs.
Analytics in logistics and distribution enables traceability and real-time visibility, which is an invaluable asset. Knowing where your goods are and being automatically alerted when there is a disruption help with developing contingency plans and with resource planning. The result is better planning, purchasing, inventory management, warehousing, distribution, and customer service.
Logistics analytics software solutions enable companies to forecast supply needs based upon real-time demand data streaming from point-of-sale systems, fleets, or warehouse sensor data. Data is gathered and combined from a wide variety of sources throughout every step of the supply chain, including the sources of raw materials, production, and distribution. Advanced analytics software can execute queries and enable rendering and visualization of spatiotemporal data, inventory, scheduling, and routing data for interactive, zero-latency data exploration and insights.
Mitigating Operational Risks
Unplanned factory or fleet maintenance requirements are sure to slow down the supply chain if we don’t anticipate them. Predictive analytics for logistics maintenance software uses data science to estimate when factory or fleet equipment might fail so that corrective maintenance can be scheduled before the point of failure.
Technologies such as acoustic, infrared, vibration analysis, and thermal imaging can be used to measure and collect operations and equipment data in real-time via remote sensor networks. These measurements are used to identify equipment vulnerabilities and predictive maintenance schedules can be implemented. Artificial Intelligence and the Internet of Things works in conjunction to automatically identify flaws and patterns in a wide variety of different factory operations and equipment functions. Automatic detection of anomalies reduces downtime and costs by quickly providing an estimate for when equipment will fail, and helps optimize supply chains in an ever-fluctuating market.
Monitoring driver behavior also helps with risk mitigation. Tools for data analytics in the logistics industry help fleet managers leverage, visualize, and truly harness the power of vehicle telematics data. An in-depth view of granular driver behavior is critical. Things like speeding, sudden braking, tailgating, seatbelt usage, or harsh shifting often go unreported, but this behavior may affect fuel use, maintenance schedules, and insurance costs.

Optimize Routing
Logistics fleet managers can use software for analytics in transportation and logistics to leverage gigabytes of location-enriched data streaming from throughout the supply chain at scale to track trucking and shipping fleets, streamline factories and production, perform route optimization, anticipate and avoid bottlenecks, and ensure prompt delivery of goods. Advanced tools for transportation analytics and logistics can handle vehicle telematics data in a way that legacy mainstream route optimization software and analysis tools cannot.
Big data analytics for logistics and transportation automatically optimizes routes based on the fastest, most cost-effective, and most efficient route of delivery. Managers can react in real-time to constantly streaming data concerning elements such as staffing, schedules, road maintenance, traffic, and weather sensors, and make agile, on-the-fly decisions based on evolving circumstances to achieve last-mile delivery efficiency.
Supply Chain Logistics Analytics Solutions
The software, tools, and platforms behind big data analytics in logistics and supply chain have advanced significantly in the last few years. As the data deluge continues growing, the tools we use have had to evolve to actually make sense of and extract value from the massive big data streams facing us every day. A critical component of supply chain optimization is being able to spot anomalies. Anomalies result in slow-downs, which have a domino effect in the chain and gum up the whole process. You can’t spot anomalies in gigabytes of data in a spreadsheet, though. Visualization is key. Data visualizations give us a dynamic, interactive, real-time view of massive datasets, enabling us to quickly understand and draw insights from the data, spot anomalies and trends, and respond with faster, better, data-driven decisions.
An advanced logistics analytics dashboard will be able to provide monitoring and reporting on important logistics KPIs. It will be able to ingest and combine big data streams from any source, in any format, at scale, in real-time, and provide clear, dynamic, and immersive visualizations that enable zero-latency exploration and insights. Augmented real-time analytics empower supply chain management teams to explore their data at the speed of curiosity.
The Future of Supply Chain Optimization
The last few years have exposed many vulnerabilities in supply chains. This is an opportunity for improvements. Decision-makers should look at their supply networks, identify vulnerabilities and inefficiencies, and invest in bringing in data scientists and in tools for data analytics in logistics and supply chain management. The future of supply chain management will see more automation and self-regulation, a greater emphasis on employee safety and satisfaction, and more demand for sustainability.
As we continue to recover from the pandemic, this crisis has alerted many decision makers that it’s time to go back to the drawing board, evaluate their procedures, and ask, “Are we operating in an ethical way? What does our supply chain network really look like? Do we have access to all of the available data that we need? Have we invested in advanced technologies that can help us stay ahead of the curve? And have we adequately equipped ourselves to be prepared for unexpected challenges?”
Dynamic logistics analytics tools for supply chain can help answer these questions and more, and help us identify problems before they ever happen. The future is filled with opportunities for logistics companies that are able to grow with the ever-evolving and expanding supply chain landscape.