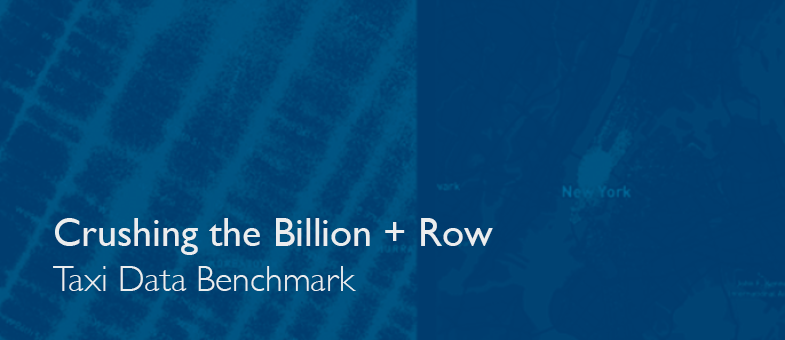
Crushing the Billion+ Row Taxi Data Benchmark
Try HeavyIQ Conversational Analytics on 400 million tweets
Download HEAVY.AI Free, a full-featured version available for use at no cost.
GET FREE LICENSEIn the dataworld, there is a particular dataset, referred to as “the taxi dataset,” that has been getting a disproportionate amount of attention lately.
The dataset in question is comprised of staggering detail (full GPS, transaction type, passenger counts, timestamps) on 1.2 billion individual taxi, limo + Uber trips from January 2009 through June 2015. Released by the New York City Taxi & Limousine Commission, the dataset became a darling of the data science set while also emerging as a popular test of database query speed.

One of the leaders on the database performance benchmarking side is Mark Litwintschik, a consultant, blogger and database fanatic from the UK. Mark has tested more than 14 different databases/configurations using the dataset since it was first released in late 2015.
All of Mark’s work to date had been on CPU based systems, the majority of which are cloud based. We were delighted when he asked to take MapD for a spin to understand if GPUs would make a difference.
Our GPU-tuned database crushed the CPU competition, running 55x faster than anything Mark had tested with the dataset leading him to say:
“For me personally, the future of BI reporting is GPU-based. The cards these benchmarks ran on are based on an architecture that's two generations old yet the query times are 55x faster in some cases than I've seen anywhere else - including large clustered CPU solutions.
MapD are a young company and it's early days with their offering but the future looks extremely bright both for them and for the world of BI.”
Mark's comments underscore our core premise, that the future of compute and visualization is GPU based.
The primary driver of this movement over the past 18 months has been deep learning and machine intelligence, but the movement is shifting downstream into more and more enterprise-grade applications – led in part by the explosion of data.
At the core, is an evolving perspective of how computing should operate — one that has a particular emphasis on massive quantities of data, machine learning, mathematics, analytics and visualization. These forces have exposed the shortcomings of the CPU while highlighting the attributes of GPUs and signal a key inflection point in computing that will ultimately permeate every enterprise, from technology giants to the local credit union.
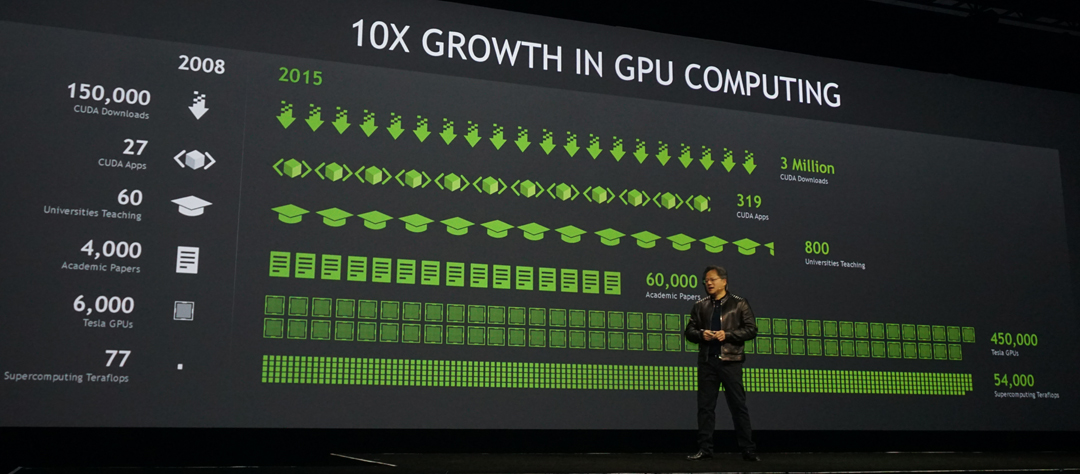
(Credit: Anshel Sag)
At 55x times faster than current “state of the art” it promises to be transformative.
Problems that appeared “too big” are now in scope.
Jobs that took “too long” will now complete instantaneously.
Visualizations that were “too complex” render in real time.
In every organization there are certain jobs that demand a different level of performance.
MapD’s capabilities across databases, visualization/BI and GIS are make it the weapon of choice for an increasing proportion of those jobs.
- The jobs that have to been done now, not overnight or even in the next half hour.
- The jobs that don’t lend themselves to downsampling.
- The jobs that require stunning visualizations on massive datasets.
- The jobs where speed-of-thought exploration results in better outcomes for the business.
We suspect you have several if not dozens of these jobs in your organization. Let’s talk about how to attack them with the right combination of hardware and software. Drop us a line at sales@mapd.com and we will put together a team of experts to talk about a POC. In the meantime, see for yourself.