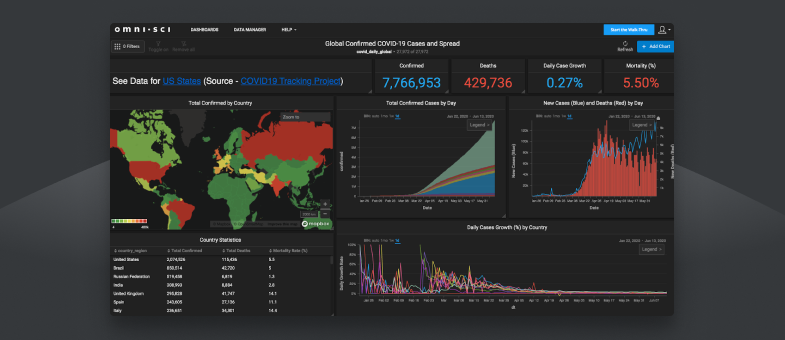
GIS Technologies in Disaster Response
Try HeavyIQ Conversational Analytics on 400 million tweets
Download HEAVY.AI Free, a full-featured version available for use at no cost.
GET FREE LICENSEIn recent years, a slew of record-breaking natural disasters has devastated communities around the world with unprecedented intensity and frequency. As this worrying trend shows no signs of slowing, the importance of investing in and incorporating advanced GIS technologies into each phase of the disaster planning and response framework cannot be overstated. While we cannot stop disasters from happening completely, GIS can mitigate risk and damages to infrastructure by helping to track, predict, and prepare for disasters, and ultimately assist in recovery and relief.
In a disaster, minutes count. The ability to quickly turn large amounts of data into actionable insights and effectively communicate the results is imperative to protect public health, minimize damage, and save lives. Here are the ways that GIS technologies are improving disaster response operations and the challenges facing disaster response teams.
Mitigation
Mitigation is a set of sustained activities undertaken in the long-term that is designed for disaster risk reduction and prevention by identifying potential hazards and their relationship to communities. Geospatial can be used to inform the modeling of risks associated with a disaster by rapidly analyzing and visualizing spatiotemporal data to better understand the impacts of these potential hazards. Disaster response jobs such as GIS Implementation Specialists are more important than ever as data streams continue to grow exponentially.
Mitigation activities may include policy changes, such as building safety code requirements; zoning restrictions regarding development in areas deemed hazardous; and benefit-cost analysis with the help of geospatial analysis. For example, utility companies can monitor power lines in real-time using data visualization tools in order to proactively identify potential maintenance needs.
A major challenge associated with disaster mitigation in the United States is public policy. The short-term, immediate tax revenue from approving development in hazardous areas, such as the wildland urban interface, which is often poorly mapped and susceptible to wildfires, may present greater appeal to policymakers than the long-term, cost-mitigating decision to preclude development altogether or to shift it elsewhere.
Public policy has the potential to mitigate disaster management and response costs, which will otherwise spill over into the private sector of insurance. Social choices dictate how we catch up with the technology that already exists. The current challenge is how we can update policies to give people the capacity to use the best available information.
Preparedness
Preparedness involves the development of an emergency operations plan with a disaster response checklist for communities by identifying data requirements, developing datasets, and sharing data across both government and non-governmental agencies. This entails short-term, collaborative activities undertaken before a disaster that ultimately enhance the readiness of inter-agency disaster response and recovery, such as the development of applications related to specific response and recovery activities, and the establishment of a dataset with common features, reporting practices, and access procedures for all potential responders.
GIS technologies aid in the development of framework and foundation data on hazards and risks, infrastructure, and the location of assets, such as generators, construction equipment, medical resources, sandbags, and shelters.
Geospatial tools like LiDAR processing are used to 3D model and test event scenarios that will ultimately aid in the development of a master scenario events list (MSEL), which enables controllers to design, test, and modify disaster response training and response plans. Models can be used to estimate the potential numbers of injuries, fatalities, and damage to infrastructure, which guides responding agencies as to when and where to safely move in.
One challenge associated with disaster preparedness is developing appropriate techniques to reach diverse communities.. GIS technologies should be used to learn from disasters quantitatively and to develop better disaster management response plans for different groups and communities of people that have been traditionally underserved. While the use of average numbers is the norm, the average should not be the focus at its present threshold in emergencies. For instance, evacuation planning does not presently, but should, take into account preparedness for the elderly, people with asthma, people with disabilities, and people with pets.
Response
This phase of responding to disasters includes the immediate activities that decrease life-threatening conditions, provide life-sustaining aid, and halt further property damage. Geospatial data is crucial in disaster response objectives such as search and rescue efforts, distribution of water and basic provisions, and the establishment of temporary power and shelters.
GIS aids in the immediate aftermath in the acquisition, processing, analysis, distribution, and conversion of images to maps of the impact area, which are overlaid with information such as locations of damages, locations of human population, locations of inventories of critical supplies, areas without power and potential timing of return of power, and maps of downed power lines and road closures. In the case of epidemics such as Covid-19, geospatial data can be used in interactive visual analytics in order to track the spread of the virus and derive insights from massive datasets.
Major challenges in the emergency response phase include quality control and information distribution. Common practices must be established among agencies regarding the integration of geospatial data into information products, such as common data reporting intervals, timestamps, and distribution methods.
Geospatial data is immense in size and the distribution of this information may be slowed down significantly due to damaged networks. Continuity planning or backup planning for disaster response communications has been an area where things have arguably gotten worse, not better. While there are recommendations, there are very few laws with teeth that require an emergency backup power source. During this time, data from ground reporting, remote sensing, geospatial models, and real-time data from in-situ monitoring may be used until imagery and verified reports are available.
Real-time analytics platforms speed up the modeling process, enabling fast visualization of data and model scenarios, which drives better mitigation, preparedness, and response decisions, and facilitates the development of an overall better disaster preparedness response program.
Recovery
The disaster recovery phase assesses the damages after a disaster occurs and contributes to the rebuilding process, educating the public, and disaster prevention practices. In the immediate aftermath, GIS helps direct short-term efforts such as tracking repairs progress, search and rescue grids, water stations, population locations, clinics’ operational status, and identifying potential temporary shelter sites.
Geospatial information also helps establish long-term recovery programs that capture and archive data from a disaster for disaster recovery analysis, including documentation of procedures and tools used for data ingestion and information distribution. Data archived during disasters is very valuable in the disaster mitigation and planning phase.
Government response to disaster varies by country, however, the functional parameters for disaster response and recovery follow the same overall guidelines laid out by the United Nations disaster response organization (UNDAC), including the National Disaster Response Framework in the United States, the Disaster Assistance Response Team in Canada, and the National Disaster Response Force in India.
Big Data and Modeling Challenges
The next generation challenge for the catastrophic disaster planning and response community is how to build nimble models that can take field information and rapidly update existing models or rerun new models. Enormous volumes of remote sensing information that may have taken five years to update 20 years ago is now continuously streaming and updated daily from constantly monitoring stations.
We have large quantities of open data and good geospatial data policies that provide access to the raw information. But how much of that information makes it into the hands of the people developing the models? We are often unable to take full advantage of the information that we're already measuring as a great deal of data is still isolated in tactical response and never makes it out to the broader disaster response community.
The biggest opportunity to improve geospatial data modeling does not require any new GIS capability to deliver, rather, it requires disaster response software, such as a big data integration platform, capable of rapid integration of continuous monitoring data and dynamic maps adaptation. There are a great deal of opportunities to learn better from the practices already in place. With proper education, the right models in place, and continuous streams of quality data, risk mapping and communication in the disaster response community will continue to improve, ultimately providing a safer environment for the people and property in the communities that they serve.